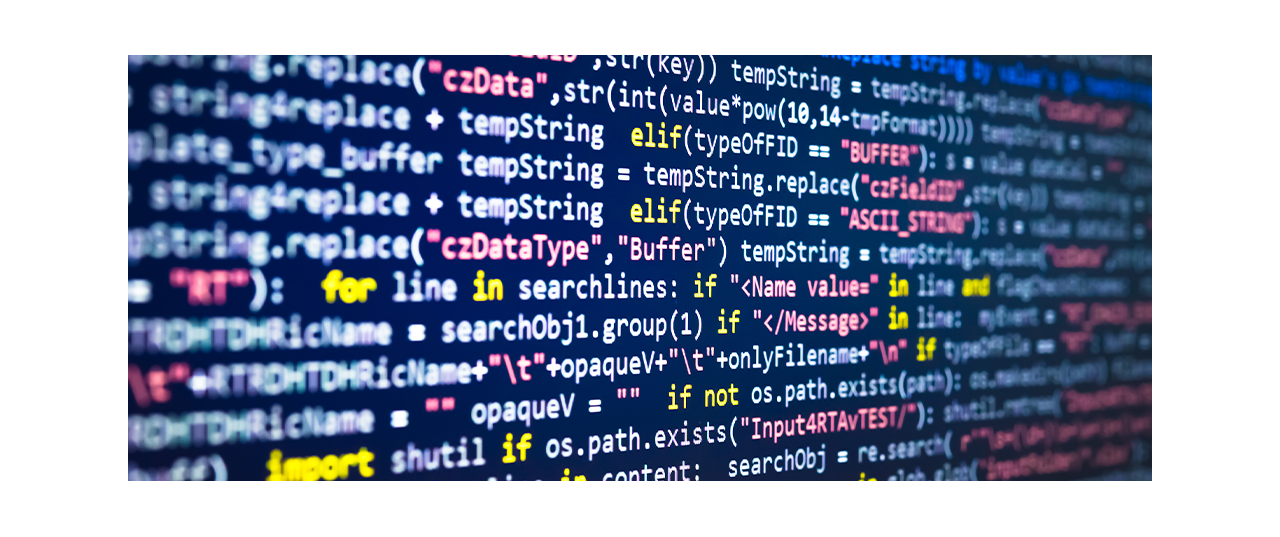
Artificial intelligence and machine learning have the potential to transform patient care, quality and outcomes. But, there are also concerns about the negative impact these technologies could have on human interaction and patient safety, including gender and racial bias.
How can an algorithm be biased?
AI is a set of tools and technologies that are put together to mimic human behavior and boost the capacity and efficiency of performing human tasks. ML is a subset of AI that automatically adapts over time based on data and end-user input. Bias can be introduced into AI and ML through human behavior and the data we generate.
There’s an assumption ML and rules-based clinical decision support applies objective data to make objective conclusions. But we're learning this is a myth—AI and ML have many points of entry that are vulnerable to bias, from inception to end use.
The ML model may be biased from the start if its assumptions are skewed. Once built, the model is tested against a large data set. If the data set is not appropriate for its intended use, the model can become biased. Bias can show up anywhere in the design of the algorithm: the types of data, how you collect it, how it’s used, how it’s tested, who it’s intended for or the question it’s asking.
As ML learns and adapts, it’s vulnerable to potentially biased input and patterns. Existing prejudices and data that reflects societal or historical inequities can result in bias being baked into the data that’s used to train an algorithm or ML model to predict outcomes.
What are the consequences of a biased algorithm?
When bias is introduced into an algorithm, certain groups can be targeted unintentionally. Gender and racial biases have been identified in commercial facial recognition systems, which are known to falsely identify Black and Asian faces 10 to 100 times more than white faces, and have more difficulty identifying women than men.
Biases in healthcare AI can perpetuate and worsen health disparities. For example,
- An AI model intended to improve clinic performance instead disproportionately decreased access to care for Black patients. Because of factors like poor access to care and perpetual lower quality of care, Black patients often mistrust the healthcare system, which can translate into higher risk for missing appointments. The patients within the higher-risk group who made their appointments therefore had longer wait times and a more unfavorable experience, increasing their no-show risk at subsequent visits.
- An algorithm that was intended to offer additional services to patients with an increased risk of disease complication used healthcare spending as a proxy for health status. This incorrectly concluded Black patients were healthier than equally sick white patients.
How do we reduce and limit bias in ML?
Algorithm design: In clinical research design, we’ve learned to mitigate bias by diversifying the groups of patients who participate in drug trials and by publishing patient demographics and study methods for transparency. The authors of a recent AMIA publication on developing reporting standards for AI in healthcare suggest four components of AI solutions that should be made transparent:
- Study population and setting: data sources, type of healthcare setting used, inclusion/exclusion criteria.
- Patient demographic characteristics: age, sex, race, socioeconomic status.
- Model architecture: model features, model output, target users.
- Model evaluation: internal and external validation methods.
Algorithm use and interpretation: ML diagnostics are just another form of a lab test. If ML algorithms provide transparent reasons for making a recommendation, then clinicians have information to validate the result or to consider how results may be biased based on a holistic view of the patient.
Adverse event detection: Consider these four ways to detect unintentional effects of AI.
- Build two different versions of a model: one with demographics included and one without. Comparing the outcomes of both models can proactively assess how much risk is due to demographics in general.
- Examine algorithm results for an unexplained minority group effect that is statistically significant when compared to the median.
- Explicitly create a quality measure of disparity that can be monitored over time. Changes in this quality measure can be investigated after a model is introduced, especially if the model continuously learns from new data.
- Follow established processes for adverse drug event reporting, which creates time frames for responding to a patient safety risk, standards for communicating the issue, resolution to affected parties and required response times for mitigation. This system might one day be adapted to include responses to unintentional bias risk.
Systemic bias: Training clinicians to be more aware of disparities is important. What might occur when clinicians encounter patients with mental illness, a history of incarceration or drug abuse, dementia, morbid obesity, economic poverty or other factors that could lead to unconscious bias? Our healthcare system, technologies and tools should be mobilized to give our clinicians more awareness of systemic bias and more time to spend with patients.
While breaking down systemic bias can be challenging, it’s important we identify and correct it in all its manifestations. This is the only way we can optimize AI and ML in healthcare
Rebecca C. Winokur, MD, MPH, is a physician executive and Tanuj K. Gupta, MD, MBA, is vice president of Cerner Intelligence, both within the Cerner Corporation.
This blog was adapted from the original version found here.